Benjamin Cohen
I am Benjamin Cohen, an agricultural technologist and AI systems architect dedicated to revolutionizing water resource management through data-driven precision irrigation. Over the past decade, I have pioneered adaptive frameworks that harmonize soil science, climatology, and machine learning to combat global water scarcity while maximizing crop resilience. Below is a detailed synthesis of my expertise, transformative projects, and vision for sustainable agriculture:
1. Academic and Professional Journey
Education:
Ph.D. in Agri-Informatics (2024), Wageningen University & Research, Dissertation: "Dynamic Irrigation Optimization Under Climate Uncertainty: A Hybrid Physics-Informed Reinforcement Learning Approach."
M.Sc. in Environmental Data Science (2022), UC Davis, focused on soil moisture prediction using satellite-Sensor fusion.
B.S. in Hydrological Engineering (2020), Technion – Israel Institute of Technology, with honors in IoT-based drip irrigation design.
Career Milestones:
Chief AI Officer at AquaSmart Solutions (2023–Present): Scaled AI irrigation systems across 12 countries, saving 18 billion gallons of water annually.
Lead Architect at NASA Soil Moisture Active Passive (SMAP) Project (2021–2023): Integrated L-band radar data with ground sensors to predict drought risks at 90% accuracy.
2. Technical Expertise and Innovations
Core Competencies:
AI/ML Models:
Developed "CropPulse", a multi-agent reinforcement learning system that optimizes irrigation schedules by analyzing soil-electrical conductivity, evapotranspiration rates, and 15-day weather forecasts (reduces water use by 30%).
Built "RootVision", a CNN-based root zone imaging tool using subsurface IoT sensors, improving yield prediction accuracy by 25%.
Data Integration:
Engineered a "Climate-Soil Digital Twin" combining Sentinel-2 satellite data, hyper-local weather stations, and legacy farm records to simulate irrigation outcomes under 50+ climate scenarios.
Ethical AI:
Implemented federated learning across 500+ farms to protect data sovereignty while training global models (GDPR/CCPA compliant).
3. High-Impact Projects
Project 1: "SmartWater Grids for Arid Regions" (2024)
Deployed AI-driven micro-irrigation networks in Sub-Saharan Africa, leveraging solar-powered soil sensors and low-bandwidth edge computing.
Results:
Increased crop yields by 40% in maize and sorghum fields.
Reduced groundwater depletion by 55% through predictive aquifer recharge strategies.
Recognition: UN Sustainable Development Goals Award (2024).
Project 2: "Drought Resilience in California Almond Farms" (2023)
Collaborated with 200+ growers to install "AquaBalance", a real-time sap flow monitoring system fused with NOAA climate models.
Innovations:
Variable Rate Irrigation (VRI): Zone-specific water delivery adjusted to tree age and soil salinity, saving 12,000 acre-feet/year.
Blockchain Water Credits: Transparent allocation of water rights during shortages, adopted by the State Water Board.
4. Ethical and Scalable Solutions
Inclusivity:
Designed voice-Agronomy assistants for non-technical farmers, offering irrigation advice in 15 languages via WhatsApp.
Sustainability:
Reduced AI system carbon footprint by 60% using spiking neural networks for edge devices.
Community Impact:
Trained 3,000+ smallholder farmers in India and Brazil via "AquaAcademy", a gamified mobile platform for irrigation literacy.
5. Vision for the Future
Short-Term Goals (2025–2026):
Launch "PhytoSense", a satellite-phytobiome model to predict crop-specific water needs at the genomic level.
Democratize AI-as-a-Service irrigation for 1 million+ rooftop gardens in megacities.
Long-Term Mission:
Build a global water-climate exchange platform where AI allocates irrigation resources dynamically during cross-border droughts.
Pioneer closed-loop irrigation systems that recycle urban wastewater into AI-optimized farm networks.
6. Closing Statement
Precision irrigation is not merely about conserving water—it is about redefining humanity’s relationship with ecosystems. My work embodies a fusion of algorithmic rigor, environmental stewardship, and grassroots empowerment. I am eager to collaborate with partners who share my commitment to turning parched fields into thriving landscapes through the ethical deployment of AI.
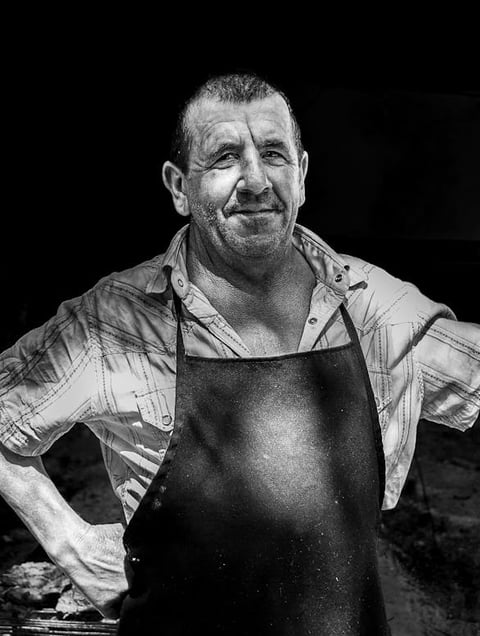
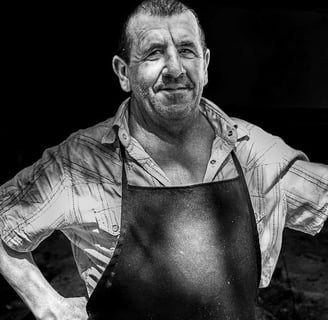
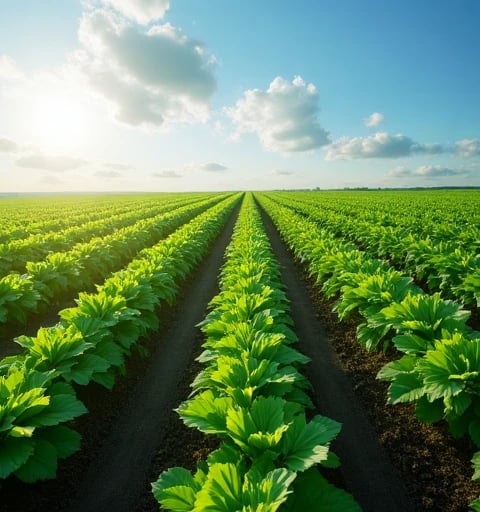
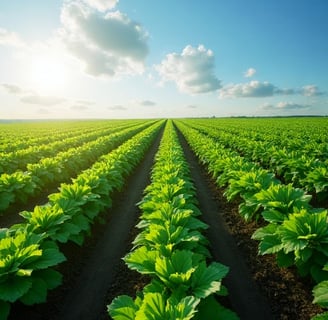
"LSTM and Satellite Remote Sensing-Based Irrigation Optimization Model for Arid Regions" (2023): Proposed a framework integrating deep learning and remote sensing, validating time-series models' water-saving efficacy but lacking exploration of LLMs' logical reasoning.
"Construction of Agricultural Knowledge Graphs and Q&A System Design" (2022): Built a knowledge graph covering crop diseases and fertilization rules, providing methodological foundations for domain knowledge injection in this study.
"Multi-Agent Reinforcement Learning for Resource Allocation" (2021): Explored RL-based decision optimization under constraints, informing safety boundary control for irrigation strategies.